High School Dropout in Proximal Context: The Triggering Role of Stressful Life Events
Abstract
Adolescents who drop out of high school experience enduring negative consequences across many domains. Yet, the circumstances triggering their departure are poorly understood. This study examined the precipitating role of recent psychosocial stressors by comparing three groups of Canadian high school students (52% boys; Mage = 16.3 years; N = 545): recent dropouts, matched at-risk students who remain in school, and average students. Results indicate that in comparison with the two other groups, dropouts were over three times more likely to have experienced recent acute stressors rated as severe by independent coders. These stressors occurred across a variety of domains. Considering the circumstances in which youth decide to drop out has implications for future research and for policy and practice.
Dropping out of high school is associated with negative individual and social consequences. In the current labor market, secondary education credentials are critical for individuals to access stable employment and earn living wages, and for increasing communities’ general living standards (Autor, 2014; Goldin & Katz, 2009). High school dropout often leads to long-term economic hardships that impair health and family functioning, which in turn can perpetuate inequalities across generations (Conger, Conger, & Martin, 2010).
In general, research focuses on long-term vulnerabilities accumulating since childhood, so little is known about the immediate circumstances triggering high school dropout (Dupéré et al., 2015). This gap is surprising both from theoretical and empirical perspectives. According to life course theories, unexpected changes and inflections in developmental trajectories, that is, “turning points,” are often brought about by external stressors that disrupt normal functioning (Elder, Shanahan, & Jennings, 2015). Empirically, research findings repeatedly show that a sizable number of dropouts abruptly and unexpectedly disengage from high school in the absence of a clear history of academic or behavior problems in elementary or middle school (e.g., Bowers & Sprott, 2012; Janosz, Le Blanc, Boulerice, & Tremblay, 2000). In these instances, very stressful and disruptive events that arise late, such as teenage motherhood, could be the determining force in dropping out. Even among youth deemed at high risk for dropout because of early trauma or persistent learning or behavior problems, such events could compound earlier risk and trigger departure, and help explain the considerable heterogeneity in outcomes among these youth (Feinstein & Peck, 2008). In short, taking into account adolescents’ current circumstances could illuminate why dropout sometimes occurs in the absence of long-term vulnerabilities, why vulnerable adolescents drop out at a specific point in time, and why only a fraction of vulnerable adolescents actually drop out.
Consistent with the notion of turning points in the life course perspective, two other, more specific theoretical models not typically applied to dropout suggest that immediate stressful circumstances could trigger negative outcomes in adolescents’ lives: the vulnerability–stress perspective and the risky decision-making perspective.
High School Dropout in a Vulnerability–Stress Perspective
Vulnerability–stress models propose that the onset of mental health problems result from an interplay between long-held vulnerabilities, such as cognitive problems or genetic or epigenetic vulnerabilities, and more contemporary exposure to stressors (Hankin & Abela, 2005). Stressors are usually defined as situations that objectively threaten physical or psychological health or well-being. They can take the form of discrete life events (e.g., the death of a parent, traumatic events) or of more enduring difficulties (e.g., a parent with a mental illness, recurring abuse; see Shonkoff et al., 2012). Numerous vulnerability–stress studies suggest that contemporary stressors are involved in the onset of a wide range of psychopathologies typically emerging in adolescence, notably depression, anxiety, behavior problems, substance abuse, schizophrenia, and eating and personality disorders (e.g., Hankin & Abela, 2005). Moreover, these studies underscore the importance of timing. Namely, they show that exposure to stressful life events tends to spike in the few months prior to the onset of a disorder (Harkness, Bruce, & Lumley, 2006). They also underscore the importance of considering cumulative exposure to stressors, as those facing multiple stressors are particularly vulnerable (Evans & Cassells, 2014).
Despite the usefulness of the vulnerability–stress perspective for understanding a wide range of problematic outcomes emerging in adolescence, educational outcomes have not been thoroughly examined through this lens. A recent review identified more than 50 vulnerability–stress studies, among which only four considered educational outcomes, and none focused specifically on dropout (Belsky & Pluess, 2009; see also Dupéré et al., 2015). Although dropout is not a psychopathology, it is detrimental to social functioning and well-being and also may be the result of both long-term vulnerability and proximal stress. The lack of attention to dropout from a vulnerability–stress perspective has stymied our understanding of the proximal processes involved, a trend that is reinforced by a parallel gap in the literature about adolescent involvement in risky behaviors.
High School Dropout in a Risky Decision-Making Perspective
Research on adolescent involvement in risky behaviors (e.g., substance abuse, dangerous driving, risky sexual practices) has reconciled two seemingly conflicting observations: adolescents can be, and often are, as rational as adults when making important decisions; however, they are also particularly prone to engage in risky behaviors with short-term benefits even when they are aware of potentially harmful long-term consequences (Steinberg, 2014). This apparent contradiction occurs because adolescents are more likely than adults to take risks only in “hot situation,” when they are under stress or experiencing strong affective impulses (Figner & Weber, 2011). Stressors appear to enhance the salience of short-term gains in comparison with long-term costs (Haushofer & Fehr, 2014), and adolescents are particularly vulnerable given their heightened sensitivity to stressors (Steinberg, 2014).
This situation could explain why adolescents quit school even if they are well aware of the costs and of the regrets that they may harbor later, notably related to restricted employment opportunities (Bridgeland, DiIulio, & Morison, 2006). In contrast with other risk behaviors, the short-term gains of dropping out may not involve immediate pleasure and peer approval (although quitting to work or to take care of a child may boost pride; e.g., Zimmer-Gembeck & Mortimer, 2006), but rather may entail avoiding punitive situations, such as academic failure or peer victimization. In that sense, dropout like other risk behaviors has short-term benefits and long-term costs, and was thus considered to be one of the key risky behaviors in early models (see Jessor, 1998, p. 4). Nonetheless, dropout has been essentially ignored in the empirical literature about how immediate circumstances shape risk-taking behaviors.
Can Psychosocial Stressors Trigger Dropout? Tentative Evidence
Consistent with the life course, vulnerability–stress and risky decision-making frameworks, a small body of empirical research suggests that stressors could play a role in precipitating dropout. This work relied on two types of designs: one that focuses exclusively on adolescents or young adults who have dropped out and another that considers general population samples.
Some studies focusing exclusively on dropouts consists of surveys about the reasons underlying youth's decision to quit school, often quite a while after their departure (e.g., Bridgeland et al., 2006). Many justify their decision, at least partly, by invoking an outside event, such as becoming a parent or needing a job to support themselves. Relatedly, detailed qualitative analysis show that dropout often occurs in the wake of a wide range of stressful circumstances, such as prolonged hospitalization, car accidents, pregnancies, teacher conflicts, peer rejection and bullying, family instability, or parental incarceration (e.g., Lessard et al., 2008; Wehlage, Rutter, Smith, Lesko, & Fernandez, 1989). They also suggest that an accumulation of stressors could be particularly problematic (America's Promise Alliance and its Center for Promise, 2014).
Despite their relevance, these studies are limited. Most importantly, because they include only dropouts, it is impossible to determine whether the identified triggers are unique to dropouts, or if they are generally shared by at-risk students, whether they drop out or not. Dropout often occurs in disadvantaged environments, and stressors identified as triggers of dropout could be as common among adolescents who drop out than among persevering schoolmates. In addition, selection bias may be at play, as dropouts’ own narratives could highlight recent events and underplay less salient but highly relevant long-term vulnerabilities, such as learning problems, underprivileged socioeconomic origins, or community concentrated disadvantage (Rumberger, 2011; Sampson, 2012).
To address such issues, a second line of studies has used general population surveys to compare stress exposure among adolescents who drop out and similar, matched peers who do not (for a review, see Dupéré et al., 2015). These studies examine the role of specific stressors emerging during high school while controlling for preexisting vulnerabilities, and some employ advanced analytic techniques to further address selection problems (e.g., Monahan, Lee, & Steinberg, 2011). These studies reveal that a variety of stressors are more common among high school students who drop out than among similar peers who do not, including school mobility, teen parenting, family instability, youth arrest, foster care placement, health problems and hospitalizations, and parental imprisonment (see Dupéré et al., 2015). These results suggest that dropout could be precipitated by such stressors.
This last set of studies also suffers from limitations, two of which are particularly noteworthy. First, they do not consider the precise timing of exposure to stressors in relation to dropout, even though studies in the vulnerability–stress perspective suggest that provoking stressors are especially likely to occur in the few months before the onset of psychopathological episodes (e.g., see Harkness et al., 2006). Knowing more about the critical period during which stressors are most likely to alter educational outcomes may help determine how long to support youth following exposure.
Second, these studies have tended to focus on a limited range of administratively recorded stressors (e.g., childbearing, parental divorce, school change) considered individually. These features are problematic, because adjustment problems can be associated with any type of significant stressor or with exposure to an accumulation of stressors (Evans & Cassells, 2014). Considering a single type of stressor in isolation, and overlooking relevant stressors that are not systematically captured in administrative records (e.g., conflicts with peers), may attenuate the relation between experiences of stress and dropping out. Relatedly, in extant studies, the association between a given stressor and dropout is typically not qualified as a function of severity, even though severe stressors are those found to trigger adjustment problems (Harkness & Monroe, 2016). These gaps are probably due in large part to the difficulty of reliably identifying all severe stressors in the life of an adolescent. Unfortunately, objective exposure cannot be assessed via easily administered self-reports because these instruments confound actual exposure to severe stressors and highly variable subjective interpretations of what counts as a severe stressor. Comprehensive interview-based instruments that avoid these pitfalls and allow for a valid assessment of severe stressors exist, but they are time consuming and thus rarely used in studies of stressor exposure (in less than 2% of studies; see Grant, Compas, Thurm, McMahon, & Gipson, 2004; Harkness & Monroe, 2016).
Objectives
The goal of this study is to examine whether recent exposure to any or to multiple severe stressors precipitate high school dropout over and above (or in interaction with) previous vulnerabilities using newly collected data from a case–control study of Canadian adolescents recruited from disadvantaged public schools. Dropouts’ stress exposure in the months preceding their departure was measured exhaustively, using a validated semistructured interview protocol that insures consistent meaning across cases. Exposure among dropouts is compared to that of similarly vulnerable schoolmates who stayed in school and of schoolmates who were not at risk for dropout. Consistent with the theoretical and empirical work reviewed, we hypothesize that (a) among dropouts, severe stressors will tend to spike in the 3 months before they quit school, whereas recent exposure should be lower among the comparison groups. Before the critical most recent 3 months, exposure should be similar among dropouts and matched at-risk students but lower among average, not-at-risk students. We also expect that (b) recent stressor exposure will remain associated with dropout even after statistically accounting for key background vulnerabilities in various ways, either directly or in interaction with these vulnerabilities.
Method
Sample and Design
Twelve public high schools participated in the project (three in 2012–2013, four in 2013–2014 and five in 2014–2015). These schools were located in and around Montreal (Canada; average school size = 1,105 students). Their average dropout rate was 36%, more than twice the provincial average, and close to the threshold of 40% used in the United States to classify high schools as “dropout factories” (Balfanz & Legters, 2004). Based on provincial official data (Ministère de l’Éducation du Loisir et du Sport [MELS], 2014), 10 of the 12 schools were located in areas of concentrated disadvantage (with high proportions of families with low-educated and nonworking parents), and two served middle to lower middle class communities; on average, the proportion of families living below Statistics Canada's poverty threshold in the 12 schools’ catchment areas was of 31%, a proportion well above the threshold of 20% used by the U.S. Census bureau to identify areas of concentrated poverty (Bishaw, 2014). The role of concentrated disadvantage is not explicitly considered in the present study but is the main object of a complementary article (Dupéré, 2016).
At the beginning of the school year, all students of at least 14 years old were invited to participate in the first phase of the study. Students who agreed (N = 6,773; participation rate = 97%) were administered a short screening questionnaire comprising a validated index measuring students’ level of risk for high school dropout (Archambault & Janosz, 2009). Then, in a second phase taking place during the rest of the school year, a subset of participants was invited to an individual interview during which stressors were assessed. The interviews were usually conducted in person, at a time and location (i.e., home, school, private room in a community center or local library) chosen by participants or, as a last resort, over the phone. All interviews were audio-recorded and conducted by trained interviewers.
The goal of the sampling design was to interview 45 adolescents in each school (or 540 overall): 15 who had recently dropped out, 15 matched at-risk schoolmates, and 15 schoolmates with an average level of risk. The schools informed the research team whenever a student dropped out, and these students were then invited for an interview. After each completed interview with a recent dropout, a matched persevering student from the same school, the same program, and the same sex was selected. Among eligible cases, the one with the closest score on the dropout risk index was selected. When there was more than one choice, the case that represented the best match in terms of age, ethnicity, family structure, and family socioeconomic status (SES; parental education, work status) was preferred. Schoolmates with scores on the risk index that were close to their school's average (calculated separately for boys and girls) were also invited to participate. They form a second, not-at-risk or “average” comparison group.
Overall, 16% of students targeted could not be reached after multiple calls. Among dropouts, 65% of those who were contacted agreed to be interviewed (participation rates among dropouts are typically below 25%; see Dupéré et al., 2015). Slightly higher participation rates were obtained among at-risk but persevering students (70%) and among average, not-at-risk students (77%). Small but significant correlations were found between nonparticipation and male gender (r = .13, p < .001) and grade retention (r = .07, p < .05), but not with other background variables (listed in Table 1).
Dropout (n = 183) | Matched at risk (n = 183) | Not at risk (n = 179) | ||||
---|---|---|---|---|---|---|
M/% | SD | M/% | SD | M/% | SD | |
Sociodemographics | ||||||
Male | 54.1 | 54.1 | 48.6 | |||
Age | 16.5a | 0.9 | 16.4b | 1.0 | 16.0a,b | 0.8 |
Immigrant status | 32.8 | 35.0 | 36.3 | |||
Visible minority | 19.1 | 24.0 | 26.8 | |||
Parental educationa | 2.5a | 1.0 | 2.6 | 0.9 | 2.7a | 1.0 |
Maternal employment | 69.4 | 70.5 | 69.8 | |||
Paternal employment | 69.4a | 80.3a | 78.2 | |||
Separated/divorced parents | 69.9a,b | 53.6a | 50.8b | |||
School-related variables | ||||||
Special education | 42.6a | 45.9b | 4.5a,b | |||
Dropout risk index (global) | 1.1a | 2.1 | 1.3b | 1.9 | −0.6a,b | 0.5 |
Dropout risk index (items) | ||||||
Retentionb | 2.3a | 1.0 | 2.3b | 1.0 | 1.4a,b | 0.6 |
Appreciation of schoolc | 2.2a | 1.0 | 2.4b | 0.8 | 2.5a,b | 0.6 |
Importance of gradesd | 3.0a | 0.8 | 3.1b | 0.6 | 3.3a,b | 0.6 |
Academic aspirationse | 4.2a | 1.2 | 4.4b | 1.4 | 4.9a,b | 1.1 |
Perceptions of gradesf | 2.7a | 0.9 | 2.7b | 0.8 | 3.0a,b | 0.6 |
Language-arts gradesg | 7.2a | 2.5 | 7.2b | 2.3 | 8.0a,b | 1.5 |
Math gradesg | 6.9a | 3.1 | 6.3a,b | 2.8 | 8.0a,b | 2.4 |
Note.
- Means and percentages sharing subscripts in each row differ significantly at p < .05, based on t tests (for means) or chi-square tests (for percentages).
- aMaximum level of education attained by one parent (1 = primary to 4 = university). bNumber of retentions (1 = none to 4 = three times or more). cAttitude toward school (1 = I do not like school at all to 4 = I like school a lot). dImportance of grades (1 = not important at all to have good grades to 4 = very important to have good grades). eAspirations (1 = no particular aspirations to 6 = university aspirations). fPerceptions of grades (1 = among the worst students to 5 = among the best students). gLanguage arts/math grades (1 = 0%–35% to 14 = 96%–100%).
A total of 545 adolescents (Mage = 16.3 years old, SD = 0.9) were interviewed, on average about 6 months after the initial screening phase (dropout events occurred throughout the year but tended to be more frequent in the winter and spring months). Table 1 presents their basic sociodemographics, along with scores on the dropout risk index (described below). As expected, both dropouts and matched at-risk students presented a less favorable profile on almost every aspect compared with normative students. As a result of the matching procedure, scores on the dropout risk index (and on individual variables comprising the index) and the proportion placed in special education were very similar among dropouts and matched at-risk students. Not surprisingly, among these two groups, average scores on the risk index were high, but large SDs indicate that there was considerable variability, with some dropouts and matched students scoring close or below average. This was predictable because some students abandon school without presenting school risk factors of dropping out (Janosz, Archambault, Morizot, & Pagani, 2008). For sociodemographics, there were no significant differences between dropouts and matched at-risk students in terms of gender, age, immigrant status, ethnicity, parental education, and maternal employment. However, dropouts were more likely to have separated parents and nonworking fathers.
Measures
Sociodemographics
To facilitate participation among this high-risk sample, no attempts were made to contact parents. Information about demographics (age, gender, ethnicity, immigration status, family structure) and family SES (parental education and working status) was obtained from adolescents during the screening phase (complemented with data obtained during the interviews in cases of partial missing data). Previous studies indicate that older adolescents (≥ 14 years old) can reliably report on some key aspects of their family's SES, including parental education, employment, and family structure, but not income, and that these reports are highly correlated with maternal reports of family income (Ensminger et al., 2000).
Vulnerability for High School Dropout
As previously mentioned, vulnerability for high school dropout was measured during the initial screening phase, when the participants were on average 15.9 years old (SD = 0.9) with an index combining answers from seven questions capturing major risk factors for dropout, including educational achievement (e.g., grades), attainment (e.g., retention), and engagement (e.g., aspirations; see Table 1). This index was validated in a large sample of high school students recruited across the province of Quebec and has good reliability and predictive validity (Archambault & Janosz, 2009). In the current sample, predictive validity and internal consistency were similarly good (area under the receiver operating characteristic (ROC) curve = 0.81, α = .76), and scores on the index predicted dropout more accurately than administrative data on failures, truancy, and suspensions (Gagnon et al., 2015).
High School Dropout
Adolescents were considered to have dropped out when they met at least one of three conditions. First, they could have filed an official notice of schooling termination before they obtained a diploma. Second, they could have decided to transfer to the adult sector (general education diploma [GED] equivalent). These students were considered dropouts because many end up not attending, and among those who do, less than one-third obtain a diploma (see Gagnon et al., 2015). Even those who become GED recipients remain more similar to dropouts than to high school graduates on a number of outcomes, including market-related ones, and are typically considered as nongraduates (Heckman, Humphries, & Kautz, 2014). Third, adolescents could have stopped attending school, without filing a notice of termination or asking for a transfer. Those who did not show up in school for at least a month without justification were thus also counted as dropouts. Because of variations across school boards regarding the management of departures and transfers, the exact prevalence of these categories is difficult to estimate, but based on a subsample (representing about a 20% of the dropouts) from one particularly meticulous school board, it is possible to estimate that these three situations, respectively, represent the main reason for dropping out for about a half, a third, and a fifth of the dropouts.
Exposure to Life Events and Chronic Difficulties
The adolescent version of the Life Events and Difficulty Schedule (LEDS) was used to measure stressors occurring over a 12-month period preceding dropout or the interview, for those still in school (Brown et al., 1992; Frank, Matty, & Anderson, 1997). The LEDS is a semistructured, interview-based instrument generally considered the gold standard for assessing psychosocial stressor exposure among both adults and adolescents (Grant et al., 2004; Harkness & Monroe, 2016). Because (to our knowledge) this study was the first one to use the LEDS in a sample of academically at-risk adolescents, minor adaptations were necessary (for additional details including psychometrics, see Dupéré et al., in press). Importantly, with the adapted LEDS, interrater reliability remained high, as did convergent validity with other sources of information (i.e., administrative records).
LEDS interview schedule
As is usually done, the interview focused on two types of stressors: discrete events (e.g., a car accident) and chronic difficulties lasting at least a month (e.g., incapacitation due to a concussion). Adolescents were asked about stressors in various domains: education, work, reproduction, housing, money, criminal or legal issues, accidents or health problems, personal relationships (with friends, family, and romantic partners), and miscellaneous. Subquestions were asked for each of these domains. In the education domain, for instance, adolescents were questioned about course failures, program or school changes, conflicts with teachers, and suspensions. Interviewers followed detailed guidelines for asking questions and used timelines to date events and chart the course of difficulties (onset, change of intensity, and termination). They did so consistently: Adherence to interview protocol was confirmed by listening to a random subset of audio-recorded interviews (Dupéré et al., in press).
LEDS coding procedure
After an interview, the interviewer prepared short vignettes (~ 150 words) objectively describing each event and difficulty experienced in the past 12 months. Using the LEDS coding manual, two research assistants blind to the adolescent's status (dropout, matched at-risk, average) independently rated stressors along various dimensions. Interrater reliability ranged from good to excellent (between .79 and .90; see Dupéré et al., in press), and any discrepancies between raters were resolved in team meetings.
A first dimension captured the classification or nature of stressors. For each stressor, one subcategory among about 100 was chosen to represent its nature. A second dimension captured the severity of the event or difficulty. For discrete events, the severity, or contextual threat, was rated on a 5-point scale (1 = marked [0.2%], 2 = moderate–high [5%], 3 = moderate–low [10%], 4 = some [38%], 5 = little [47%]). For chronic difficulties, severity was captured using a 6-point scale (1 = high-marked [0.1%], 2 low-marked [3%], 3 = moderate–high [17%], 4 = moderate–low [41%], 5 = mild [31%], 6 = very mild [7%]). Because the severity of difficulties can fluctuate over time, they were classified according to the most severe rating attained during the past 12 months. A last dimension represents the level of independence. Totally independent stressors are out of the control of the participant and not influenced by his or her behavior (e.g., grandmother death). In contrast, dependent events are shaped by the participant's behavior, and he or she plays a role in their emergence or escalation (e.g., interpersonal conflicts). Some events fall in between, in the sense that they are probably largely independent but could have been influenced by the participant in some indirect or small ways (e.g., the relapse of a bipolar parent). Accordingly, independence was rated along a 3-point scale (1 = totally independent event [25%], 2 = probably independent [12%], 3 = dependent [63%]).
For analytic purposes, we distinguish between exposure to severe, moderate, and mild events or difficulties (Monroe, Slavich, Torres, & Gotlib, 2007). Following established LEDS cutoffs, severe events and difficulties were defined as those receiving moderate–high threat ratings or above. Events and difficulties receiving moderate–low threat ratings were classified as moderate, and remaining ones with lower threat ratings as mild. For difficulties, two additional aspects were considered. Previous research shows that difficulties are particularly problematic not only when they are severe but also when they are prolonged and ongoing (see Brown & Harris, 1978; Monroe et al., 2007). Thus, only difficulties that lasted at least 6 months and that were still present around the time of the interview were examined (for a similar approach, see Shih, Eberhart, Hammen, & Brennan, 2006).
Results
Descriptive Statistics About Exposure to Events and Difficulties
Percentage exposed to severe, moderate, and mild stressors were computed among dropouts and comparison groups (matched at-risk and average schoolmates). When relevant, distinctions were made between exposure to one versus multiple stressors. Chi-square tests (df = 2, N = 545) were conducted to test for statistical significance; standard errors were bootstrapped to account for data clustering (students within schools; Cameron & Miller, 2015).
Events
The 12-month period covered by the interview was separated into four equal 3-month (13 weeks) periods, thereafter referred to as quarters. Mild events were frequent during all quarters in all three groups, and no significant differences emerged (full results available upon request). For this reason, mild events were not considered further.
In contrast, for severe events, significant differences were found between dropouts and the two other groups but only in the most recent (fourth) quarter (χ2 = 26.5, p < .001). During this quarter, exposure spiked among dropouts (see Figure 1A) and reached 19.7%, whereas the rate was three to four times lower among matched at-risk and average students, among whom it was 6.0% and 5.0%, respectively. During the other, earlier quarters, exposure among dropouts was much lower and not significantly different from the two other groups. When distinguishing between recent (fourth quarter) exposure to one event only and to two or more (2+) events, results showed that exposure to 2+ events was 12 times higher among dropouts (6.0%) than among matched at-risk (0.5%) and average (0.6%) schoolmates (χ2 = 15.5, p < .001), whereas exposure to one event only was about three times higher among dropouts (13.7%) than among the other two groups (5.5%, 4.5%; χ2 = 12.8, p < .01).
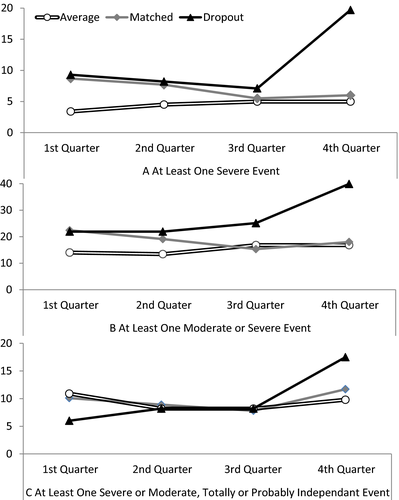
Although moderate events were generally more frequent than severe ones, the pattern of between-group differences was comparable to that observed for severe events: the proportion of adolescents with at least one moderate event did not significantly different across groups except during the fourth quarter (χ2 = 17.0, p < .001). At this point, the proportion was about twice as high among dropouts (29.5%) compared with their matched at-risk (14.8%) and average (14.5%) peers. These differences between groups remained essentially proportional when considering exposure to only one versus 2+ moderate events, with exposure in both cases being about twice as high among dropouts than among the other groups (full results available upon request).
A similar pattern was apparent when exposure to at least one significant stressful event (severe or moderate) was considered, with significantly higher exposure among dropouts than among the two other groups, but only in the fourth, most recent quarter (χ2 = 32.9, p < .001). Figure 1B shows the highest exposure among dropouts in this quarter (39.9%), whereas exposure was about half that among matched at-risk (18.0%) and average schoolmates (16.8%).
The moderate to severe events experienced by dropouts in the fourth quarter were distributed among a variety of domains and categories. About 24% of these events were school-related (12.8% for conflicts with school personnel, 11.2% for other disruptions such as school change, failure). Remaining nonschool-related events included family conflicts or crises (17.6%), peer-related problems (18.3%; about evenly divided between conflicts with peers and problems in romantic relationships), legal problems (15.2%; such as arrests, court appearance as a victim or defendant), and “other” problems (24%; about evenly distributed between health and residual, miscellaneous problems). All of these five broad types of events were significantly higher (at p < .05) among dropouts than among at least one of the comparison groups, with chi-square values ranging between 7.9 and 14.4, except for the “other” residual category. For the latter, exposure was about twice as high among dropouts (10.9%) than among matched (5.5%) and average (6.7%) schoolmates, but this difference did not reach statistical significance (χ2 = 4.2, p = .12).
Severe Chronic Ongoing Difficulties
Mild and moderate difficulties were frequent among the three groups of adolescents, and the rate of exposure was not significantly higher among dropouts than among the two other groups (full results available upon request). For this reason, moderate and mild difficulties were not further considered.
By contrast, severe difficulties were not equally distributed (χ2 = 22.5, p < .001). The proportion of adolescents exposed was higher among dropouts (51.9%) than among matched at-risk (29.5%) and average (33.0%) students. Further analysis showed that exposure to a single severe difficulty was not significantly more frequent among dropouts than among the two other groups (χ2 = 1.7, p = .42). Rather, it was exposure to 2+ severe difficulties that was particularly high among dropouts (25.7%) as compared with matched at-risk (8.7%) and average (11.2%) schoolmates (χ2 = 23.7, p < .001).
As for events, the severe difficulties experienced by dropouts were distributed among a variety of domains and categories. About a third of difficulties were school related (23% involved protracted course failure, 6% involved chronic conflicts with school personnel), whereas a quarter (25%) involved recurring family conflicts. Problems with peers and in romantic relationships (e.g., chronic victimization, social isolation) represented about 16% of severe difficulties. Recurring criminal or legal problems were rare (2%) and thus were considered along with chronic health problems (18%, distributed about evenly between the participants themselves and significant others) and other, miscellaneous problems (11%) into a fourth residual “other” category. Chronic school- and family-related difficulties were significantly (at p < .001) higher among dropouts than among at least one of the comparison groups, with chi-square values of 33.96 and 13.05, respectively. For peer-related and “other” difficulties, exposure tended to be higher among dropouts than among the other groups, but chi-square did not quite reach significance (with χ2 = 3.9 and 5.7, respectively, and ps = .056 and .144). However, when these nonschool- and nonfamily-related difficulties were collapsed into a single category, between-group differences reached statistical significance (χ2 = 7.6, p < .05).
Multiple Logistic Regressions
Although the matching procedure was generally successful, dropout and at-risk adolescents were not identical (e.g., dropouts were more likely to have nonworking fathers, see Table 1). To take these differences into account, multiple logistic regressions were used to predict dropout based on acute and chronic stress exposure while controlling for key available background characteristics. Again, standard errors were bootstrapped to account for data clustering (students within schools; Cameron & Miller, 2015).
Multiple logistic regression analyses focused on the matched dropout and at-risk adolescents (n = 366). Average adolescents, who were recruited to provide a normative benchmark, were not matched to adolescents of the other two groups and tended to have different backgrounds (see Table 1). Accordingly, average participants were not included in the multiple regression analyses because they did not represent an adequate comparison group for gauging the role of proximal stressors, over and above preexisting vulnerabilities. Among dropouts and matched at-risk students, these preexisting vulnerabilities were captured with the variables presented in Table 1, and with new control variables representing the number of severe and moderate events experienced in the first three quarters (3–12 months prior to dropout or prior the interview for nondropouts). These variables represent participants’ general tendency to experience stressful events, based on exposure prior to the focal fourth quarter.
A number of overlapping variables were used to represent stressors in the descriptive analyses (coded to distinguish the severity, broad domain, and number of stressors). To avoid redundancy in the final full models, preliminary logistic regressions focusing only on recent stressors were conducted to identify the stressor variables that had independent, nonredundant associations with dropout. For events and difficulties, the stressor variable with the strongest chi-square in the descriptive analyses was inserted first while gradually incorporating those with lower chi-square values until the added variables no longer made independently significant contributions. For events, this procedure resulted in the selection of two variables: exposure to at least one recent severe event and to at least one recent moderate event. For difficulties, only one variable was independently associated with dropout and retained: exposure to 2+ severe difficulties. Preliminary tests were also conducted to probe for interactions between acute events and chronic difficulties and vulnerabilities captured by the dropout risk index, but no trend emerged. Thus, these interactions were not included in the final logistic regression models.
The results of the final logistic regression model including the retained stressor variables alongside the full set of control variables are presented in Table 2 (Model 1). They show that recent exposure (fourth quarter) to both severe and moderate events was significantly associated with students’ greater odds of dropping out, but earlier exposure (first to third quarter) to either types of event was not. In addition, the odds of dropping out were significantly higher among students exposed to two or more severe chronic difficulties that were ongoing around the time of the interview. Effects sizes were larger for recent exposure to severe events and difficulties (adjusted OR = 3.46 and 3.43, respectively) than to moderate events (adjusted OR = 2.05).
Robustness checks | ||||||
---|---|---|---|---|---|---|
Model 1 Unweighted regression | Model 2 IPTW-weighted regression | Model 3 Alternative measure of exposure | ||||
OR | 95% CI | OR | 95% CI | OR | 95% CI | |
Sociodemographics | ||||||
Male | 0.99 | [0.57, 1.72] | 0.93 | [0.52, 1.67] | 1.04 | [0.61, 1.77] |
Age | 1.42** | [1.08, 1.88] | 2.13*** | [1.51, 3.01] | 1.38* | [1.03, 1.85] |
Immigrant status | 1.48 | [0.62, 3.55] | 0.81 | [0.33, 1.99] | 1.35 | [0.57, 3.16] |
Visible minority | 0.74 | [0.29, 1.89] | 1.50 | [0.41, 5.57] | 0.74 | [0.29, 1.88] |
Parental education | 0.95 | [0.71, 1.28] | 0.90 | [0.64, 1.28] | 0.96 | [0.72, 1.28] |
Maternal employment | 1.04 | [0.57, 1.89] | 0.76 | [0.40, 1.45] | 1.05 | [0.58, 1.91] |
Paternal employment | 0.66 | [0.34, 1.26] | 1.36 | [0.74, 2.48] | 0.68 | [0.38, 1.23] |
Separated/divorced parents | 2.00** | [1.16, 3.45] | 1.59 | [0.88, 2.91] | 2.01* | [1.18, 3.43] |
Dropout risk | ||||||
Special education | 0.86 | [0.47, 1.58] | 1.31 | [0.69, 2.49] | 0.88 | [0.50, 1.54] |
Dropout risk index (global) | 0.43 | [0.04, 4.71] | 0.09* | [0.01, 0.64] | 0.42 | [0.04, 4.19] |
Dropout risk index (items) | ||||||
Retention | 2.02 | [0.13, 30.2] | 9.10* | [1.04, 79.6] | 2.02 | [0.15, 27.1] |
Appreciation of school | 0.56 | [0.20, 1.56] | 0.29** | [0.12, 0.70] | 0.57 | [0.21, 1.53] |
Importance of grades | 0.90 | [0.45, 1.78] | 0.58† | [0.32, 1.04] | 0.84 | [0.43, 1.64] |
Academic aspirations | 0.76 | [0.49, 1.18] | 0.49** | [0.32, 0.76] | 0.76 | [0.48, 1.18] |
Perceptions of grades | 0.77 | [0.37, 1.60] | 0.72 | [0.40, 1.30] | 0.76 | [0.38, 1.52] |
Language-arts grades | 0.82 | [0.46, 1.45] | 0.60* | [0.38, 0.94] | 0.83 | [0.48, 1.43] |
Math grades | 0.91 | [0.52, 1.59] | 0.55* | [0.35, 0.86] | 0.90 | [0.52, 1.54] |
Nb of life events first to third quarter | ||||||
Nb of severe events | 0.93 | [0.56, 1.54] | 1.01 | [0.74, 1.37] | 1.01 | [0.64, 1.60] |
Nb of moderate events | 0.99 | [0.73, 1.35] | 0.83† | [0.68, 1.02] | 1.05 | [0.78, 1.43] |
Stress exposure in the fourth quarter | ||||||
≥ 1 severe ev. | 3.46** | [1.30, 9.21] | 5.33*** | [3.06, 9.30] | ||
≥ 1 moderate ev. | 2.05* | [1.05, 4.01] | 1.39 | [0.76, 2.56] | ||
≥ 2 severe chronic difficulty | 3.43** | [1.46, 8.04] | 2.65** | [1.37, 5.16] | 3.92** | [1.77, 8.68] |
Stress exposure in the fourth quarter to independent ev. | ||||||
≥ 1 severe/moderate independent ev. | 2.00† | [0.88, 4.53] |
Note.
- Ev. = stressful life event; Nb = number.
- *p < .05. **p < .01. ***p < .001. †p < .10.
Robustness Checks
Robustness checks of our main findings were conducted because this study is based on observational data, meaning that the association between recent exposure to severe stressors and dropout could reflect selection issues. Namely, students who experienced recent severe events could be different from their unexposed peers on a number of measured and unmeasured characteristics not fully accounted for by the matching procedure and the use of logistic regression models. They could, for instance, be particularly impulsive and prone to behavior problems. Such unmeasured traits could both increase the likelihood of exposure to stressful events and of dropout and thus spuriously inflate the relation between these last two variables.
To address these issues, propensity score analyses were used to further adjust for background differences between those who were exposed to severe events in the fourth quarter and those who were not (Guo & Fraser, 2010). Propensity scores are meant to capture the fact that some individuals are more likely than others to be exposed to a given etiological agent (in the present case, stressful events) and to adjust the analyses accordingly.
In a first step, participants’ propensity to be exposed to severe events was established via a logistic regression analysis predicting exposure in the fourth quarter. The parameters obtained in this regression analysis were then used to calculate predicted values for each participant. These predicted values represent each participant's propensity score, that is, their propensity to have been recently exposed to severe stressors based on their profile on the variables included in the equation. The variables included in the logistic regression to establish propensity scores were not limited to basic sociodemographic and school-based vulnerabilities listed in Table 1. Variables capturing past exposure to stressors were also incorporated and were those most strongly associated with recent exposure (see Table S1) and appear to capture propensity for exposure at least to some extent.
In a second step, we used the formula for estimating the average treatment effect in the treated (Austin & Stuart, 2015) to transform propensity scores into inverse probability of treatment weights (IPTW). Balance checks were performed to ascertain that these weights successfully rebalanced students who were recently exposed to a severe event and those who were not, in terms of family background characteristics, past schooling outcomes, and past exposure to stressors (see Table S1).
Incorporating IPTW in the logistic regression models did not substantially change the results for recent exposure to severe events, as this variable remained associated with dropout, again with a large effect size reflected by an OR > 3 (see Table 2, Model 2). In the IPTW models, other measures of recent stress exposure (exposure to moderate events and to severe ongoing chronic difficulties) were less strongly or no longer associated with dropout, suggesting that selection processes may be at play for these variables.
As a final robustness check, we considered only recent (fourth quarter) independent, “act-of-god”-like events that were unlikely to have been caused or exacerbated by the adolescents’ characteristics (e.g., personality issues), that is, severe or moderate events (combined because of power issues) rated as independent or probably independent (for a similar approach, see Harkness et al., 2006). Considering only this category of events did not alter the pattern of findings of the descriptive (Figure 1C; chi-square for differences in the fourth quarter between dropouts and matched schoolmates = 4.5, p < .05) or regression (Table 2, model 3) analyses, although in this latter case the OR was only marginally significant (p = .067).
Discussion
It is commonly assumed that dropping out of school is the result of a protracted process of academic failure and adjustment problems starting to unfold as soon as children enter school and even before (Dupéré et al., 2015). In this study, we proposed that other, short-term processes occurring late in students’ schooling career are also relevant. Namely, we hypothesized that recent stressors play a role in precipitating high school dropout over and above, or in interaction with, preexisting vulnerabilities.
In terms of our first hypothesis, the findings showed as expected that exposure to severe stressful events spiked among dropouts in the few months prior to their departure and that during this period it was much higher than among matched at-risk and average schoolmates. In other periods, exposure among dropout was not significantly higher than among matched at-risk schoolmates. These results echo previous vulnerability–stress findings indicating that exposure to severe stressors tend to spike in the 3 months prior to the onset of various adjustment problems (Harkness et al., 2006). Also in line with the vulnerability–stress literature, we found that dropping out of school in stressful circumstances was quite common: approximately 40% of students who dropped out did so in a context where they were recently exposed to at least one moderately or severely stressful event. These events were not confined to a single domain; rather they occurred in a range of areas related to school, family, peers, romantic relationships, and courts of law. Finally, descriptive results showed, again as expected (see Evans & Cassells, 2014), that exposure to two or more severe stressful events was particularly problematic, but this result should be interpreted with caution due to low base rates.
In terms of our second hypothesis, the results showed that recent exposure to severe stressors remained directly and strongly (with adjusted ORs > 3) associated with dropout, even after accounting for preexisting vulnerabilities and general propensity to experience stress. The role of severe stressors was not limited to adolescents who were already at very high risk, as no interaction was found between exposure to recent events and preexisting vulnerabilities. However, it is important to acknowledge that participants were recruited from highly disadvantaged schools with high dropout rates and were all vulnerable in this respect.
Further supporting our second hypothesis are findings showing that even events that were unlikely to have been caused by adolescents themselves, and thus to reflect their own predispositions, were associated with dropout. For instance, some dropped out not long after the suicide of a close friend, a hospitalization for blood poisoning, the arrival of an unstable psychotic relative in an already overcrowded home, or the firing from her job of a bipolar mother following a relapse. These findings echo others studies highlighting that events outside adolescents’ control can have acute negative consequences for their educational trajectories (DeLuca, Clampet-Lundquist, & Edin, 2016; Dupéré et al., 2015).
Events that are outside of adolescents’ control provide a useful approach for addressing selection issues. However, it does not mean that events for which adolescents bear at least part of the responsibility are irrelevant, if only because such events are comparatively much more frequent. In fact, over 50% of the recent moderate and severe events experienced by dropouts involved conflicts with family members, peers, romantic partners, or law enforcement officers, in which adolescents necessarily played a role. Similarly, a number of well-controlled studies show that events that are not random act-of-god-like events, such as becoming a parent, can result in an increased risk of dropout (Ashcraft, Fernández-Val, & Lang, 2013). In short, whether or not adolescents are responsible, experiencing a severe stressful event can mark adolescents’ entrance into a period of vulnerability.
Theoretical Implications
For at least 40 years, prominent theoreticians of dropout have hinted that proximal circumstances could be a part of the dropout process (Finn, 1989; Tinto, 1975; Wehlage et al., 1989); however, their models did not formally include or delineate this specific aspect. This gap was bridged in a recent integrative, life course framework of high school dropout that explicitly proposed an etiological role for proximal stressors (Dupéré et al., 2015). This framework incorporated insights into broad developmental theories underscoring how circumstances at one point in time can shape developmental outcomes. The results of our study support this framework, and more broadly, the general developmental theories that inspired it.
The results are first and foremost consistent with the life course idea that even though developmental trajectories tend to be relatively stable, ruptures and inflections are also an inherent part of development (Elder et al., 2015; Rutter, 1996). The results illustrate this idea of “turning points” occurring in the face of changing circumstances, as severe stressors appeared to trigger dropout, an important rupture in adolescents’ educational trajectories. The results are also consistent with vulnerability–stress models proposing that proximal stressors can trigger the onset of a pathological episode, along with long-term preexisting risks (Belsky & Pluess, 2009; Hankin & Abela, 2005). Similarly, the results are aligned with theoretical models and empirical studies on risk-taking behaviors, as this work underscores how immediate situational cues (e.g., the presence of peers) can increase the likelihood of engaging in a given risk behavior regardless of preexisting risks (e.g., impulsivity; Figner & Weber, 2011; Steinberg, 2014).
The findings also provide an illustration of the developmental psychopathology broad principle of equifinality (Cicchetti & Rogosch, 1996). This principle, also echoed in social-ecological models of student engagement (Lawson & Lawson, 2013), dictates that a given developmental outcome can be the endpoint of a number of different pathways. Consistent with this principle, the findings suggest that dropout does not solely result from pathways characterized by protracted problems and long-held vulnerabilities. Stressors emerging late in high school are also important, both because they can precipitate dropout among those already at risk or unexpectedly inflect trajectories among those with comparatively moderate levels of risk.
Practical and Policy Implications
Practitioners and researchers interested in dropout prevention are aware of the heterogeneity of pathways leading to dropout, and many have rejected “one size fits all” approaches in favor of integrated ones covering a range of academic and social needs (Bloom, Thompson, & Ivry, 2010; Janosz et al., 2008; Lawson & van Veen, 2016). Our findings support this view, by highlighting the need to address not only early emerging, persistent vulnerabilities such as learning problems but also the immediate circumstances in which dropout occurs (for similar arguments, see DeLuca et al., 2016; Steinberg, 2014). The findings are also relevant for school policies regarding conflict management, as the impact of stressors acted out on the school premises such as peer and teacher conflicts can be exacerbated by disciplinary actions that intensify alienation or stress, such as out-of-school suspensions (Noltemeyer, Ward, & Mcloughlin, 2015).
School personnel need to be aware of the precipitating role of stressors: First to be prepared to provide extra help to high-risk students facing a crisis, and second to better identify students who are temporarily at risk even though they were never seen as problematic. Of course, their ability to know when students are under acute stress depends on students’ voluntary disclosure and on the staff's willingness to receive sensitive personal information. Because teachers are in daily contact with students, they are in principle well positioned to build bonds and perceive vicissitudes in their students’ life. In practice, however, high school teachers are typically content specialists who lecture in front of large, rotating classes and are unlikely to be attuned to all of their students’ changing needs, particularly in nonacademic spheres. This difficulty is compounded in more disadvantaged schools, where students’ needs are greater and where many teachers have insufficient training, experience, resources, or time (e.g., due to temporary assignments, turnover; see Murray & Malmgren, 2005). In this context, it might be unrealistic to expect teachers to focus on issues beyond basic instruction.
Nevertheless, when trained and properly supported by mental health professionals who can take over difficult cases, teachers can build trusting relationships with students and help them cope with academic as well as nonacademic challenges, even in disadvantaged high schools (Franklin, Kim, Ryan, Kelly, & Montgomery, 2012). A number of strategies could be implemented to strengthen teachers’ and school professionals’ capacity to detect and address their students’ changing needs following exposure to severe stressors. The provision of regularly updated and readily available information about late arrivals or uneven attendance can be a useful first step, as upticks could facilitate the identification of students who are under stress at a given moment and in need of extra attention (Christenson & Thurlow, 2004). To this end, new technologies designed to improve the detection of fluctuating risks, and to allow for “just-in-time” interventions delivered when vulnerability is at its peak, could be helpful (Lagoa, Bekiroglu, Lanza, & Murphy, 2014).
However, warning systems focusing only on attendance or other administrative data might not be sensitive enough to identify all at-risk students in time, for instance, because some of them may go through stressful circumstances, but nevertheless attend school regularly until they dropout (Dupéré et al., 2015). A more comprehensive detection approach, like the ones used to detect exposure to recent stressful and traumatic events among students (Ko et al., 2008), might thus prove necessary. Interestingly, such comprehensive approaches to detection would be coherent with the philosophy of multiservice schools designed to respond to the multifaceted needs of struggling students from disadvantaged communities (Lawson & van Veen, 2016; Lawson & Masyn, 2015).
Strengths and Limitations
The strengths of the study derive in large part from its sampling and assessment procedures. Sampling was specifically designed to uncover the proximal processes leading to dropout. Dropouts were contacted right after they had left school, as opposed to years later (a common practice in other studies), so as to reduce memory bias. In addition, a credible comparison group was created by carefully matching each dropout with a similar peer, selected among more than 6,700 students screened for this purpose. In addition, efforts were invested during the screening and interview phases to obtain high participation rates among a notoriously recalcitrant group: at-risk adolescents attending disadvantaged schools. Finally, a comparatively large sample was interviewed, considering the demands of the assessment protocol.
In terms of measurement strategy, we precisely identified when students dropped out. Carefully considering timing is crucial to understand the proximal mechanisms involved, but few dropout studies do so. Many studies use broad, retrospective definitions of dropouts and related categories (e.g., early school leavers) that are imprecise with regard to timing. For instance, the common practice of defining early school leavers as young adults (18–24 years old) without a high school diploma who are no longer in training lumps those who dropped out recently and those who dropped out years ago (see Gillies & Mifsud, 2016). Moreover, some students who quit without a diploma and reenrolled only years later may not be counted as early school leavers. Strategies that clearly identify who drops out and when such as the one used in this study are thus needed to provide information about what can be done when and for whom to support resilience (Dale, 2010). More attention to timing could contribute to the development of a better understanding of when certain disruptive situations tend to occur in the course of students’ careers and when extra vigilance is warranted.
Another strength is the assessment of exposure to stressors with the LEDS, an instrument recommended for its reliability, validity, and comprehensiveness (Harkness & Monroe, 2016). However, because the LEDS is time consuming, it is rarely used, and when it is, samples tend to be relatively small. To our knowledge, the present study is the first one to comprehensively assess stressor exposure among high school dropouts with this instrument. In large part thanks to this innovation, this study offers original findings underscoring the etiological relevance of exposure to any severe stressor during the few months preceding dropout.
Despite these strengths, a number of limitations should be noted. First, we sought to have a near perfect participation rate during the screening procedure. Achieving this goal required tradeoffs in terms of the number of questions asked and of sources consulted. For instance, no attempts were made to obtain information from parents (e.g., about income), and information on adolescents’ early academic and behavioral history was collected indirectly, via cumulative measures (e.g., of grade retentions). At-risk students could only be matched to dropouts on measured characteristics. Accordingly, unmeasured differences, for instance, in terms of family income, could be responsible for the observed association between severe stress and dropout. This is unlikely, however, because family background seem to influence educational outcomes through proximal processes that were measured (e.g., aspirations, grades, retentions; Polidano, Hanel, & Buddelmeyer, 2013) and the association between stressors and dropout remained strong and robust even when these processes were accounted for.
Second, even if a state-of-the art instrument was used to assess stressors, some problems may remain. For instance, dropouts could retrospectively have exaggerated stressful life circumstances to provide an a posteriori justification for their decision to quit school. This situation is unlikely, however. When possible, we found convergence between adolescents’ reports and administrative data, for instance, with regard to school suspensions (Dupéré et al., in press). Also, participants were not asked to provide a rationale for their decision to drop out. Rather, they were simply asked about the challenging situations they had experienced in the past year, without any reference to dropout. In addition, if dropouts were exaggerating the stressfulness of their circumstances, it is unclear why they would have done so only for a specific 3-month period and not for the whole year covered by the interview. Finally, although recent minor events are recalled more easily than older ones, such recency biases are not observed for severe events, as these tend to be recalled accurately over long periods of time. In fact, in the present study, as in previous LEDS studies (Brown & Harris, 1978), the base rate for severe event tends to be generally constant across time in general population groups.
Third, even though the participants were recruited in 12 high schools located in a variety of communities, all of the schools were from one region, thereby potentially limiting generalizability. It is worth noting, in this regard, that the likelihood that our findings are generalizable is increased by the fact the association between contemporary stressors and dropout was observed in all our participating schools. In addition, it could be argued that our findings provide a detailed description of a phenomenon (i.e., the link between contemporary stressors and dropout) that has been detected in studies conducted in a wide range of western countries, including the United States and Canada (for a review, see Dupéré et al., 2015).
Relatedly, even though comparatively high participation rates were obtained for the interview phase of the study, a significant proportion of dropouts could not be reached or refused to participate. Even if nonparticipation correlated with only two background characteristics (male gender and grade retentions) and weakly so, the results may not adequately represent the experiences of those dropouts who were not in the sample. Namely, assuming that those under very high stress are particularly hard to reach and to recruit, exposure to recent severe stressors among dropouts could be even more common than estimated. In addition, because the participants were recruited from disadvantaged schools, the results may not represent the experiences of youth who drop out in more advantaged contexts.
Conclusion
In sum, high school dropout is a highly problematic outcome that is associated with heavy and enduring individual and social costs. For this reason, preventing dropout is a key goal for a number of public and private organizations (see America's Promise Alliance and its Center for Promise, 2014). This study offers new insights into the proximal context in which dropout occurs. Importantly, the results show that high school students who are exposed to severe acute stressors are momentarily vulnerable to dropping out. Accordingly, timely and strategic interventions could help these students cope with crises and find alternative solutions that do not result in abandoning school.