Abstract
Chaotic phenomena are not part of standard curricula, although this subject offers several interesting aspects which can help students better understand basic features of science. A central observation is that even simple physical systems, if chaotic, are unpredictable, just like the weather. We present the principles applied when developing a freely available short interactive material and outline the material itself. By the means of this material students can become acquainted with the elements of chaos science and also learn that the regular motions taught in physics lessons are the exceptions rather than the rule since a slight modification of the set-up (e.g. letting the suspension point of a pendulum move periodically) converts the motion to chaotic. The material can be used in both classroom and online teaching.

Original content from this work may be used under the terms of the Creative Commons Attribution 4.0 license. Any further distribution of this work must maintain attribution to the author(s) and the title of the work, journal citation and DOI.
1. Introduction
The aim of this article is to find an approach making students acquainted with chaos-related phenomena via their own observations and experience. Below, we summarize current approaches which are common and stimulate interest in chaos physics but are not effective for students studying physics because the science applied to get the results is missing.
- An introduction based on videos showing only numerical simulations of differential equations might be not convincing (see e.g. [1]).
- A treatment based on the history of science is appealing and illuminating (see e.g. [2]) but cannot be made condensed enough for a short material.
- Astronomy offers nice instances of chaotic phenomena [3], but a systematic investigation of conservative chaos with its hierarchical structure is too much at a first encounter.
- Program packages which can be run just by typing in the right-hand side of the differential equation, and also offer visualization options for chaos [4], might be useful for students interested in informatics, but not for public education.
- A number of popular demo experiments of chaos (like e.g. magnetic or double pendula) illustrates the complexity of the motion but, in the lack of any driving, dissipation takes over and all motion stops ultimately. Such experiments thus illustrate the transient form of chaos [5] whose understanding requires advanced concepts. The first encounter with chaos is better to be based on driven systems 1 .
- Fractals are aesthetically appealing companions of chaos. In relation to the phenomenon of mixing and stirring they can successfully be incorporated in chaos teaching (marbling or other handicraft techniques, see e.g. [6]), there is, however, a danger that they receive too much attention, and less focus remains on physics 2 . A short material should therefore treat fractals in a low key.
2. Mixing and unpredictability
Motivated by these observations, a teaching material has been developed during the last years [9]. Chaos phenomenon is well suited for becoming acquainted with unpredictability. The mere fact that an initially concentrated amount of material becomes rapidly distributed in a large domain (like, e.g. salt in a dough when kneading) is an essential observation. The very first question is related to the picture in figure 1 where a dye distribution is shown after a few movements of stirring. The question is if the reader can determine the initial dye pattern from such a photograph? The fact that this is impossible is a hint on the unpredictability of mixing processes
Figure 1. Fluorescein on the surface of glycerol after a few stirring movements with a pencil (original size 5 × 5 cm).
Download figure:
Standard image High-resolution imageFigure 2 shows a picture of drifting ice along the coast of Kamchatka, resulting in the same phenomena on a global scale.
Figure 2. Satellite image (NASA) on the continental scale: drifting ice along the coast of Kamchatka http://efluids.com/efluids/gallery/gallery_pages/Vallis.jsp, [17].
Download figure:
Standard image High-resolution imageWe learn that the dispersion of any material, among others the spreading of pollutants, is unpredictable, and at this point the student can also add: the spreading of pollution in air or water is a chaotic process. Mixing is considered useful in the household (e.g. mixers), is a basic element of environmental pollution, and its convoluted pattern makes (among others) the protection of our environment a real challenge.
These examples also illustrate the interdisciplinary aspects of chaos, providing a hint both on its relations to art and on its relevance in geosciences. Furthermore, one becomes acquainted with fractal-like patterns here, without any relation to chaotic attractors.
The core material is devoted to the discussion of the seemingly contradictory main features of chaos [8]: (a) long-lasting motion of relatively simple systems which is irregular in time, (b) sensitive to initial conditions: in other words, unpredictable, (c) structured in an appropriate view exhibiting fractal patterns, (d) in spite of the impossibility of the long-term prediction of individual histories, the probability of describing the permitted outcomes is fully predictable (a property much less widely known). Via sequences of questions, the material helps students realize that the listed characteristics (a)–(d) are all present in chaos simultaneously.
3. The core material
The core material is composed of four modules devoted to the aforementioned basic properties of chaos. Two modules can be completed in about one standard 45 min teaching period. The presentation concentrates, as much as possible, on a home-made experiment and video records taken with it. At the end of each of these modules, the readers are offered a short quiz to provide immediate feedback on their progress, including the correct answer where a question is answered incorrectly. An efficiency better than 80% is honoured by a 'smiley face'.
3.1. Temporal irregularity
Students get acquainted with the experimental setup to be used: a physical pendulum represented by a ruler whose suspension axis is moved periodically by means of an electric motor, a wiper from a car (see figure 3, left panel). The dynamics is unavoidably dissipative since friction and air drag (even if weak) are present. Students can observe the pendulum motion in video records. It is striking to see (not only at the first time) that pieces of the motion are never repeated. One automatically tries to extract some rules, but this is hopeless, the motion is aperiodic (see figure 3, right panel).
Figure 3. Left panel: screenshot from the first video with the experimental setup, a driven pendulum (the green bar is a 30 cm ruler). Right panel: time series of the angular velocity of the ruler measured by means of a sensor.
Download figure:
Standard image High-resolution imageThe motion of the usual, undriven pendulum is well known, and its swinging repeats itself periodically (as long as friction remains negligible). Such regular motions taught in physics lessons are the exceptions rather than the rule: one also learns that a slight modification of the setups (as the moving suspension point here) converts regular motions to be chaotic.
3.2. Unpredictability
This feature is rather surprising in the context of mechanics, but one immediately accepts its relevance when looking at a video taken with two identical pendula suspended on the same axis, just somewhat shifted along it, driven by the same motor. The motions become spectacularly different within a few seconds as figure 4 illustrates.
Figure 4. Screenshot from the video exhibiting the motion of two identical pendula (green and blue rulers) subjected to the same driving. Although, the rulers are from the same producer and thus technically identical, and the driving is also the same, when they start from the same initial condition, after about 15 s of identical behavior, their motion suddenly becomes completely different (the snapshot shown is taken after 30 s). The blurred picture of the green ruler illustrates that the velocities are rather different, too.
Download figure:
Standard image High-resolution imageEven if the initial states of the rulers appear to be the same, there are always minor differences. It is these differences which become magnified in time: small uncertainties lead to dramatically different outcomes. This observation illustrates the term 'dependence on initial conditions', but also motivates such motions to be called unpredictable. The term 'butterfly effect', often used in the popular culture (see e.g. [2]), expresses exactly the same property.
To deepen the concept, we also show the result of a numerical simulation in which 11 pendula are followed with the same driving but slightly different initial conditions (figure 5, upper panel). When comparing this with a forecast diagram (lower panel) we realize that our simple driven pendulum appears to be as unpredictable as the weather.
Figure 5. Illustration of unpredictability. Upper panel: the angular velocity time series of 11 driven pendula starting from nearly identical states, represented by different colours. Lower panel: an ensemble simulation (of 30 members) in a weather forecast for Budapest, downloaded from [10]. The teaching material directs students to this webpage where anyone can generate the weather prediction in the form of the lower panel for their city on the day of visiting the page.
Download figure:
Standard image High-resolution imageA comparison with the case of a regular pendulum is worth making here, too: the motion of two pendula with nearly identical initial conditions would stay close over long periods of time. Regular motions are not sensitive to initial conditions, are predictable, the butterfly effect is not present in such cases.
4. Further analysis of core material data
This is where we show that further analysis of the data can lead to the recognition of more delicate, but equally important, additional features.
4.1. Structured patterns in special views
Chaos is related to complex patterns but these become visible only in properly chosen samplings. A natural choice is taking snapshots after full periods of the driving, when e.g. the suspension point is at its furthest position to the right. At these instances the position and velocity of the pendulum are recorded, are plotted as a point of a plane, and this is repeated over a long time. The material presents measurement results of the single-pendulum setup extracted via a digital angle sensor. The initially seemingly random appearance of points on the position-velocity plane starts, after some time, to accumulate in certain regions, and avoiding certain others. It is remarkable to see that a kind of order appears in this view: a huge number of points are allowed to occur during the chaotic motion, but many others are forbidden. The set of points appearing in this representation provide a characteristics of the long-term dynamics, called a chaotic attractor (see figure 6).
Figure 6. Chaotic attractor of the driven pendulum in a numerical simulation: points representing the angle of the pendulum and its angular velocity are plotted at integer multiples of the driving period. Nearby points merge into green filaments. Note that there is a huge number of points permitted, but much more, the ones in the black regions, cannot occur in the long-term motion. The permitted states are arranged along a folded, interwoven filamentary pattern. The magnifications on the right illustrate that filamentarity is present on all scales, the pattern is a fractal.
Download figure:
Standard image High-resolution imageChaos is thus not complete disorder; it is found to be structured when observed from appropriate views. It is important to realize that this is a consequence of a definite rule being in the background, namely Newton's law! One thus learns that chaos is not noise (as also illustrated by the smooth graph of the time series in the right panel of figure 3).
High precision numerical simulations of the driven pendulum are also shown to provide a really intricate view of the chaotic attractor, as also illustrated by figure 6. (To see such fine details, a long simulation of at least 10 000 periods is needed which can be written in any standard programing language. One just needs to mark the points visited on the position-velocity plane).
To grasp how much different regular motions are, imagine a long-lasting periodic motion. This would be represented by a single dot in the sampling used. Chaos is thus infinitely more complicated than regular motion.
4.2. Predictability with probabilities
The long-term motion related to a chaotic attractor can be properly characterized by asking about the probability with respect to which a permitted state (e.g. the state with the fastest possible rotation of the ruler) occurs among all possible motions. The probability with which different regions of the attractor of the chaotic pendulum is visited can numerically be determined and leads to the striking form seen in figure 7. (The procedure is similar to that applied when creating figure 6, but one also marks how often a point (a pixel) is visited, and this number is plotted on the perpendicular axis.) We have to digest that the existence of this probability distribution follows from the Newtonian equation of motion!
Figure 7. Probability distribution associated with the chaotic attractor of the driven pendulum. Probability is represented by the height of the thin columns. The distribution is rather uneven: the probability of a given state might be completely different from that of the neighboring state. This fine dependence is a property of chaos-related probabilities.
Download figure:
Standard image High-resolution imageThe analysis of chaotic systems teaches us that from a statistical point of view the motion developing on the attractor can be described with full accuracy. Even if individual motions are unpredictable, the statistical properties of all possible motions can be predicted! The somewhat pessimistic view of interpreting the 'butterfly effect' as not being able to be sure of anything, should be counterbalanced by the potentially perfect ability for a statistical characterization of chaos 3 . We are then not surprised by seeing weather [10] (or most recently even climatic [11]) forecasts moving in the direction of providing likelihoods rather than precise predicted data—this is the proper treatment of such problems.
After reviewing the main properties, two remarks might be in order:
- (a)The view suggested by the science of chaos is analog with that of quantum mechanics: trajectories are ill-defined and a probabilistic description should be applied to properly handle the problem. In chaos, however, this holds in a classical, macroscopic world!
- (b)Climate is characterized by typical features, averages, which follow immediately if the probability distribution is known. Since climatic averages can, in principle, be given for the future, we can say that climate is predictable. In a weather forecast, however, we intend to foresee the continuation of a single recorded past, and this is impossible (for more than a few days), weather should be considered unpredictable.
5. Branching off materials
Students can follow the linear main path of the course, a motivated reader can, however, find extra questions to answer. Two more substantial branching off materials are also added which can be accessed either from the webpage or also independently.
Since numerical simulations occur in [9], we decided to develop an additional webpage [12] that allows students to monitor motions by themselves. This introductory material is based solely on the use of Excel worksheets, and has been found to be useful (in non-chaotic contexts, too) in different classes from grade 9 upwards. By means of the last worksheets, they have the opportunity to change, say the fourth digit, of the initial position of a chaotic system. Upon clicking on 'return', the graphs characterizing the motion belonging to the previous initial condition suddenly change their shape on the screen: the 'butterfly effect' is in action in the students' own simulation.
Another side branch is related to the spreading of volcanic ash in the atmosphere (see figure 8). A freely accessible program [13, 14] can be downloaded on the student's computer. The simulation is based on meteorological wind data measured in a certain period over the Globe, and generates the path of a large number of small, spherical particles of the typical density of volcanic ash in this wind field. Students can choose the location of their 'own' eruption anywhere and follow its evolution. The primary message is that the initially localized ash cloud becomes strongly stretched and folded soon. The arising pattern (figure 8) is similar in character to that of figures 1 and 2, just the time dependence can also be seen in this case. Unpredictability, or the butterfly effect, is well visible here on a global scale: the largest initial distance between ash particles of at most 1 km has grown up to about 10 000 km in 10 days.
Figure 8. Instantaneous distribution of the volcanic ash (all colored dots) above Eurasia arising from a localized eruption within Europe 10 days earlier. Atmospheric dispersion of pollutants is a chaotic process. Color indicates the height: blue meaning high, red low latitudes, generated by means of [13].
Download figure:
Standard image High-resolution image6. Conclusions
Even if the use of Internet resources is rather natural for both students and teachers, few of these resources support learning materials in sufficient depth. The physics of chaotic phenomena appears to represent a gap to be filled, in particular in terms of materials that can be processed within a few teaching periods of public education. Our preliminary experience shows that within such a short time, students are able to understand that chaoticity is accompanied with features appearing very different and surprising at first sight, but all originating from the fact that unpredictable behavior is common even in the realm of simple physical systems.
The material is not only suitable for independent learning, teachers may also use it as a resource. Perhaps not only physics teachers, since there is an emerging view in education theory [15, 16], according to which the outcome of the teaching process itself is often unpredictable, and thus chaos-like. Therefore, an elementary knowledge of chaos theory might be beneficial for anyone participating in education.
Acknowledgments
The paper is devoted to the memory of Márton Gruiz, coauthor of [8] and collaborator in lecturing and developing teaching materials on chaos in the period of 2000–2017, who shaped the basis of the view and material presented here.
Special thanks are due to A Tél for the completion of the experimental setup and for carrying out the sensor-related measurement, and to B Tél for producing the screenshots used in this paper. The author thanks Z Neufeld and M Gruiz for numerical simulations, M Vincze for contributing to video recordings and providing useful suggestions on how to present the probabilistic aspects, T Weidinger for calling the author's attention to webpage [10], I Bajkó for her participation in the selection of the questions in the quizzes, and F Urban for technical assistance in developing the webpages. The advices of K Guseva on structuring the material, and of A Teiermayer, B Tél and M Vincze concerning phrasing are also acknowledged. Constructive suggestions of an anonymous reviewer to consolidate the text are highly appreciated.
The project is funded by the Content Pedagogy Research Program of the Hungarian Academy of Sciences, and by the NKFIH Office under Grant K-125171.
Data availability statement
The data that support the findings of this study are openly available at the following URL/DOI: http://theorphys.elte.hu/fiztan/chaos/.
Footnotes
- 1
Where a continuous energy injection compensates dissipation, in the long run at least.
- 2
In particular, most of the popular fractal examples (like e.g. Sierpinski gasket, Koch curve, tree-like structures [7]) have nothing to do with chaos since the invertible character of the equations of motion restricts the fractal types occurring in chaos physics, and Cantor filaments remain the only major option [8].
- 3
Regular motions are also fully predictable in terms of probabilities but their distributions are rather trivial: single peaks.
Biographies
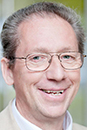
Tamas Tel has been active in chaos-related research since 1982. In the last 25 years, he regularly offered special courses on chaos for trainee teachers and PhD students. The material of these was published in [8]. He is head of the MTA-ELTE Physics Education Research Group (2016-2021).